Now & Next: Deep Learning
by Hugh Williams on 11th Oct 2017 in News
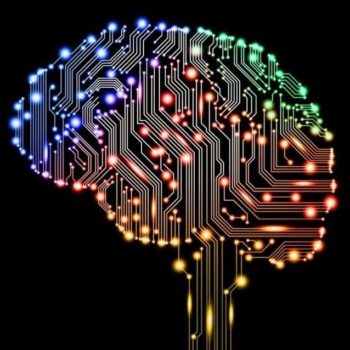
As our reliance on artificial intelligence (AI) grows, so do expectations that computers will come up with solutions for increasingly complex problems. In order to sate our appetite for AI, deep learning is coming to the fore. This edition of ExchangeWire’s Now & Next looks at why deep learning is proving more important than machine learning, its role within advertising, and whether its presence is a positive, or, as some believe, could lead to human extinction.
Brothers, but not twins
While deep learning and machine learning are both members of the artificial intelligence family, they are significantly different. As the picture suggests, we can think of deep learning as machine learning’s heavier, wiser sibling.
Although both are capable of making decisions based on data, deep learning systems use more data, which is filtered through a greater number of layers (or neural systems). This means that when a decision is finally reached, it is more informed and more accurate. The more data we feed deep learning systems, the smarter they become. The combination of graphics processing units (GPUs) and CPUs help expedite this process. Machine learning systems simply cannot make quick enough sense of this amount of data.
A clearer customer picture
With programmatic ads relying on the collection and interpretation of consumer data to stand out, the successful application of deep learning systems has the potential to revolutionise the industry.
These processes are already helping retargeting. Deep learning algorithms can improve the user experience by analysing behaviour and predicting the probabilities of specific purchase events more accurately than a human (or a more simplistic program) ever could. This helps divert ad spend to sites and occasions where purchase is most likely. It is the power of deep learning that enables these systems to make rational decisions without human input.
Beyond retargeting, deep learning is being used in shops to create improved customer lifetime value through automatic feature learning. Feature learning, whereby machines recognise vision, audio, or text patterns, traditionally has to be designed by humans, which takes time and means machines can only recognise a finite amount of detail. Automatic feature learning, on the other hand, means the systems are self-taught in this area.
Together with in-store sensors (which have the capabilities to track new versus repeat customers, visit frequency and duration, and store navigation), automatic feature learning’s ability to recognise patterns is enabling marketers to focus on high-value customers, while minimising engagement with unprofitable customers.
Robots creating masterpieces
It’s clear that deep learning (and AI in general) is already having, and will continue to have, a profound effect on programmatic. What’s not so clear are the ramifications it could have when it comes to making creative decisions.
At present, this hurdle remains unconquered. While enabling deep learning systems to make decisions based on a huge amount of data is one thing, enabling them to mimic the creative design process is quite another. This is where human-computer cooperation could play a role.
Human-computer cooperation derives from the idea that, while we as humans are limited in terms of our ability to deal with large data volumes, we can exhibit startling levels of creativity within our thought. The opposite can be said of machines.
Deep learning algorithms have the potential to monitor the purchase patterns (online and offline), content consumption (by genre, and whether the majority of consumption is in text or video form), and lifestyle habits of customers. Together with data about the type and size of ad units available on particular sites, these algorithms could then recommend to creatives the optimal ad to create engagement or drive purchase for this customer. This is where the creative team will take over, designing the ad and feeding it back into the algorithm to place on the best site to reach this consumer.
However, it is by no means inconceivable that the creative process itself won’t one day be subsumed by algorithms. All it will take is an ability to replicate and edit images that it has previously seen, rather than solely recognise them. Given the capabilities of deep learning and the vast amounts of money being pumped into AI (startups received USD$5bn [£3.88bn] in 2016 – up 1681% since 2011), why couldn’t this happen?
In fact, the powers that be at Google had already been asking themselves similar questions when they created DeepDream. Instead of only recognising images and being able to classify them, DeepDream is intended to take an original image (such as the photo on the left), and make a new image from it (the finished product on the right).
If the system sees a jellyfish that it thinks has a slight resemblance to the shape of a platypus, the deep learning technology will make a new image which looks more like a platypus. Therefore, the next time the image is passed through DeepDream, it will have a stronger representation to a platypus, meaning the third iteration created by the technology will be an even more platypus-esq jellyfish image, until we are ultimately left with an image similar to the one on the right.
Creating the enemy
Despite the efficiencies that deep learning can create within programmatic, this wouldn’t be a real AI article if I didn’t at least touch on the possibility that by nurturing intelligent machines, we might be setting ourselves up for extinction. However farfetched this may seem, some of the world’s sharpest minds, including Stephen Hawking and Elon Musk (as well as numerous sci-fi films), think that by enabling machines to become more intelligent than ourselves, we are endangering our existence.
Hawking explained how if deep learning enables machines to reach their full intelligence, the gap between their intelligence and our own will be similar to the difference in intelligence between humans and snails.
In this instance, extinction won’t be malicious, but practical. In 2015, Hawking said: “The real risk with AI isn't malice but competence. A super-intelligent AI will be extremely good at accomplishing its goals, and if those goals aren't aligned with ours, we're in trouble. You're probably not an evil ant-hater who steps on ants out of malice, but if you're in charge of a hydroelectric green energy project and there's an anthill in the region to be flooded, too bad for the ants. Let's not place humanity in the position of those ants.”
If we are to heed these warnings, then the future of deep learning will be forever limited by human self-preservation. Though it may never reach its full potential, we will see an increase in human-computer cooperation, letting deep-learning-enabled computers do all the leg work, while humans make the ultimate decisions. However, if human curiosity to develop more advanced technology gets the better of us, the potential to wipe out the human race must be evaluated alongside a deep learning algorithm’s ability to tackle some of the world’s most pressing issues.
Follow ExchangeWire