How AI Can Be Employed by Brands to Bolster Customer Engagement & Sales
by Romany Reagan on 15th Jan 2018 in News
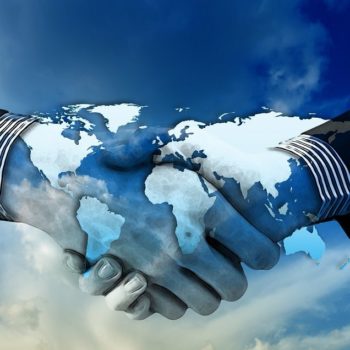
As an industry, we have been highly focused on applying a scientific approach to relationship marketing; but it is important to realise that relationships start long before someone presses the ‘buy’ button. In this piece, Pini Yakuel (pictured below), CEO of Optimove, discusses how businesses can use AI tools to improve customer engagement.
One of the major challenges that businesses face is bridging the gap between the art of customer engagement and the science of data. CRM practitioners face the daily challenge of leveraging their data to make customer communications more effective and help them to increase sales.
We are increasingly seeing marketers utilising different technologies in order to maximise the effectiveness of their communications; and as a result many businesses of all sizes are exploring the ways in which advancements, such as AI and machine learning, can give them a competitive edge.
Personalised messaging needs to be a top priority for businesses that wish to thrive amidst the noise of digital marketing. By tapping into AI and machine learning, businesses can remove the guesswork involved in the most difficult aspects of personalisation and CRM.
Truly effective personalisation results in improved customer engagement, loyalty, and spend; but only 30% of customers feel that they are getting their desired level of personalisation. This highlights that, while many businesses are putting a greater emphasis on data collection and testing, they are not effectively employing the data to implement truly tailored messaging.
Machine learning shouldn’t be seen as a way to replace the marketing or the CRM team, but rather as a tool to turbo charge the effectiveness of their campaigns. It is the first real tool to enable truly granular marketing, promising more engaged customers, better conversion rates, and powerful, agile marketing. The technology can optimise campaigns by:
Dropping underperforming actions: When one campaign action is clearly losing to others, it will recommend dropping the underperforming action and readjusting the existing actions.
Switch to self-optimising campaigns: Once enough data has been collected on a certain campaign, the technology will recommend turning on its self-optimising feature to automatically decide the correct customer mix for each campaign action.
Focus on key target groups: In situations where only a small subsegment reacts well to a campaign, its best to split the campaign and create a new campaign for the underperforming segments.
Verification: Verify that all campaigns are scientifically measured for uplift by recommending a control group in order to measure the true impact of the campaign.
One of the main ways that machine learning can be applied is to plan and enhance every customer interaction, through continuous tests that constantly optimise the performance of customer communications. Generalisation can have a detrimental effect on customer engagement. Until the ideal match between every campaign and customer segment can be found, businesses are potentially missing out on improving brand awareness and increasing sales opportunities. This is where continuous testing and optimisation bridges the gap, as it enables businesses to maximise the effectiveness of their messaging.
Ultimately, testing enables businesses to collect data on customer preferences. This information can then be employed to create highly effective AI-based customer marketing campaigns by analysing a business’s customer base, identifying the wants and needs of customer microsegments, and matching them with the most relevant offer.
The more granular the customer group, the better the offer can be tailored to customers’ specific needs and wants, creating a higher level of engagement. Segmenting customers into distinct personas on a daily basis is a highly valuable practice that enables marketers to understand what makes their customers tick, and how to best interact with them by categorising customers into different ‘lifecycle stages’, which represent the different phases that customers move through during their journey with your business. The stages can then be employed to provide a granular and far more accurate representation of the customer database, which can then be used to create targeted marketing which will improve customer engagement.
It is essential to remember that, whilst customer segmentation can lay the basic foundation for personalisation, true segmentation goes beyond basic insights such as: lifecycle stages, recency, frequency, monetary value (RFM), and product preference. A multidimensional approach is required to enable businesses to observe different data perspectives, allowing for a more complete understanding of your customer.
Due to the perceived complexity of machine learning, a number of businesses are still lagging behind, as it is often believed that data scientists and developers need to be involved in order to create effective AI tools. However, it is more of a case of businesses rethinking how they distribute their budget between acquisition and retention. Whilst in the past, the vast majority of a business’s budget was invested in acquisition, we are now seeing forward-thinking companies shifting towards a balance between acquisition and retention, in order to make the most of their existing customer base.
As an industry, we have been highly focused on applying a scientific approach to relationship marketing; but it is important to realise that relationships start long before someone presses the ‘buy’ button. Although not perfect, AI-driven predictive analytics make the most accurate predictions possible, allowing marketers to maximise the impact of their efforts, ultimately leading to improved customer engagement and increased profits.
Artificial IntelligenceDigital MarketingDisplay
Follow ExchangeWire