Measuring the ‘Mesut Özil’ Clicks of a Complex Customer Journey
by Sonja Kroll on 4th Apr 2016 in News
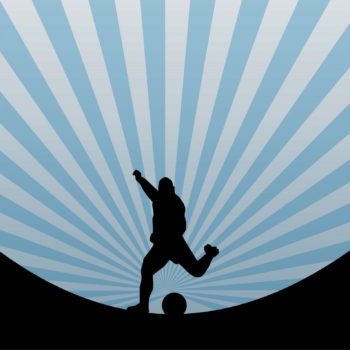
Winning the English Premier League isn’t solely dependent on the last goal of the season. This is a given for anyone mildly interested in football. Adam Baker, MD, Sociomantic UK, (pictured below), uses the analogy with football to take an in-depth look at the customer journey on the way to a purchase – and what the individual phases of this journey actually mean for marketers.
For those with a waning interest in Arsenal FC, or this present season — it’s understood that Danny Welbeck’s recent goal against Leicester City didn’t automatically grant him the title 'man of the match'. The honours went to Mesut Özil for his free-kick, which ultimately assisted Welbeck’s deciding goal – leading to a 2:1 win for Arsenal.
Given the current league standings, football is a sore topic for this self-confessed Gooner, but an apt analogy for smart online marketing attribution. Whilst Mesut Özil isn’t near the top 10 Premier League goals scorers this season, he is still regarded as one of the league’s most important players. If each player out on the pitch is given credit for their overall contribution to the match, or if each fixture won (or drawn) is attributed points, I wonder why we don’t work harder to apply the same logic to our online marketing attribution.
Why do so many advertisers still place so much emphasis on that last-click to purchase —the last foot to propel the ball into the net?
It’s not to say that I don’t understand the appeal of last-click attribution. Of course it’s clear: this model is easy to grasp and easier to implement than its more mature attribution counterparts.
In our connected world, however, a competing brand is never more than a mobile search away. So, it’s important that advertisers understand how the different points of contact influence the decision to buy (or not to buy). By adopting a ‘customer journey’ attribution model, which takes into account numerous touch points rather than simply the one closest to conversion, advertisers will get a deeper and clearer understanding of their customers’ paths to purchase — and where to spend their marketing pounds.
Once, the journey might have entailed walking down the grocery aisle. Now, it could include anything from engaging with a mobile banner, to searching for a brand or product name, to cashing in on a voucher code and walking down the grocery aisle, basket in tow. Because the customer’s path to purchase has transformed so dramatically, it is only logical that the attribution model mirror its changes.
Admittedly, this is easier said than done.
It requires defining how valuable each touchpoint — each assist — is in achieving the desired outcome.
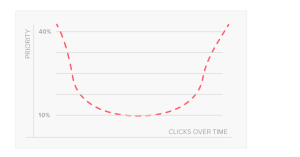
Example of an inverted bell curve attribution model
For example, a retailer with a long sales cycle may allocate the highest percentage of credit for a given sale to the first touchpoint. By defining a business-specific ‘attribution curve’ which assigns a weight to the various touchpoints based on where they occur in the journey, advertisers can then divvy up the effective media costs across the various touchpoints.
Perhaps an online shop specialising in sunglasses decides to assign higher value to the first and last click of their customer’s journey, while assigning relatively lower value to clicks occurring in the middle of the journey. Let’s say the first click was generated by SEO. After scrolling through the ‘Wayfarers’ category and a newsletter registration in exchange for a 10% discount on her first purchase, the customer navigates away from the site. A few days later, a retargeting banner offers related product recommendations and our shopper is intrigued by the different types of shades. She clicks through, has another browse through the category before finally adding a pair to her shopping basket. During checkout, she remembers her first-purchase discount. Heading over to her email inbox, she finds the promotional email and clicks through to claim her discount. Success.
In this case, attribution could be broken down as such:
– 40% attributed to SEO (first click)
– 20% attributed to display retargeting (assisting click)
– 40% attributed to email voucher (conversion click)
Mind, this was a fairly simple example. It becomes doubly more difficult for marketers taking into account brand engagements that occur not only across multiple devices, but also during offline interactions.
The Cross-Device Conundrum
Handling an average of five devices, today’s Brits are an internet-savvy bunch, only surpassed by one more device on average by our American counterparts. With this many connected gadgets, it’s all the more important to track engagement across devices for complete customer journey attribution.
But how can advertisers recognise customers across their different devices? And after having done so, how do advertisers resolve which are the more valuable interactions?
There are two camps when it comes to determining how to match customer profiles across devices: probabilistic versus deterministic.
The probabilistic school of thought is statistical. It necessitates analysing swathes of interactive data — connected networks, visited locations, visited websites, to name a few— before nosing out patterns and pairing likely matches. By comparison, deterministic user-matching hinges on using unique identifiers (e.g., email address or customer ID) to tie up people’s interactions across connected devices.
Probabilistic matching has the benefit of scale, but often faces criticism for lack of accuracy. Meanwhile, deterministic matching affords advertisers the opportunity to implement consistent segmentation and personalisation strategies across devices, but can face limits of scale because, in many cases, it requires customer login. But, that login can provide a joined-up view of a customer’s actions across devices and, ultimately, the ability to measure the effects of media exposure for each logged-in device.
Connecting the Dots Between Offline and Online
Equally, if not more, challenging is multi-channel attribution, in which marketers must measure the impact of digital media on offline sales, and offline media on digital sales. Typically, the success of non-direct offline campaigns, such TV or radio, is measured largely by correlation analysis — for example, how much does website traffic increase around the same time as the campaign? Whilst not an exact science, this method does allow the advertiser to continually learn more insights from how offline affects online behaviour.
But the buck doesn’t have to stop at correlation analysis. Offline sales measurement practices, such as loyalty cards or offline transactions tied to email addresses (e-receipts = attribution gold), can in turn help advertisers to tie offline purchases more easily to online influences.
So, how does the attribution work?
When an offline sale is tracked and attached to a unique ID, such as a loyalty card number or email address, the advertiser can attribute the sale to the user’s online advertising touchpoints that happened immediately prior to the sale. This method can even lead to the calculation of a higher CLV (because shoppers who shop both offline and online are likely to have a higher overall value), and completely new possibilities for reactivation campaigns, personalised marketing and more.
Let’s say a customer checks out SunglassesShop.com several times, having been directed to the site via different online marketing channels — search, email, and display. Alhough she spends quite some time browsing the latest Wayfarers, she doesn’t make a purchase. However, she’s exhibited strong purchase intent by logging into the site with her username and password, placing her into an ‘existing customer’ segment. The next day, she heads to the Sunglasses Shop in the mall nearby her flat. There, she purchases the pair she had been eyeing up online. The retailer then emails her receipt to the same email address used to log into SunglassesShop.com. Since the sale can be attributed to the same ID, the advertiser can now take into account the assisting digital touchpoints that contributed to this offline purchase.
The Best Model is a Custom Model
Selecting the right attribution model, implementing and testing that model, adjusting online marketing targets every couple of weeks — digital marketing attribution has never been easy, and it’s not getting easier. But where some see challenges, there is certainly opportunity to innovate.
Most advertisers know they need to crack the multi-device conundrum in order to maintain a sustainable marketing budget, rather than spend inefficiently across the different channels and devices.
If advertisers are armed with an efficient and aggressive digital marketing strategy, it would be a shame — or worse, wasteful of precious marketing budget — to ignore interactions with the various marketing campaigns, channels, and vendors that have helped generate the desired action: a purchase.
I’d liken it to disregarding Aaron Ramsey or Alexis Sánchez, or Mesut Özil’s 18 (near-record breaking) assists this season. It’d be insane to brush aside these contributions during a football season, so why do it during a customer’s journey?
AdvertiserattributionDisplayProgrammatic
Follow ExchangeWire