The Future of Measurement: Q&A with Eoin O'Neill, Chief Technical Officer, Tug
by Grace Dillon on 28th Feb 2022 in News
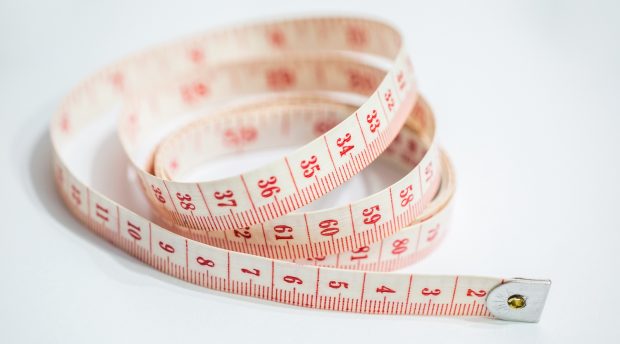
In this exclusive Q&A, Eoin O'Neill, chief technical officer at Tug, talks to ExchangeWire about the changes taking place in digital marketing measurement and what advertisers can do to adapt to these.
How do you see digital marketing measurement changing in the next year?
Major changes are needed in mindset and approach. Almost since the start, digital marketing has taken a relatively simplistic full-funnel approach to measuring consumer journeys and advertising performance. This has been heavily influenced by the perception of online media as providing better measurability and precision than offline media, especially for paid efforts.
This was considered a positive evolution that brought marketing closer to an exact science. A prime example being frequent comparisons between detailed digital accuracy and linear TV, which traditionally only allowed advertisers to drive general brand awareness. Now, however, shifts across the measurement landscape are making deep granularity hard to sustain.
To take measurement to the next level, the industry must move towards a more nuanced understanding of user behaviour, intent, and engagement, using known outcomes. One way to think of it is the difference between applying physics on earth — assessing defined and tangible objects — and how things are measured in space (with scientists tapping into varied data sources, running tests and creating models for how the universe probably works). In short, it’s about shifting from exactitude to likelihood, backed by smart insight.
What is driving this shift?
While developing precise methods for tracking individuals across their digital footprint has fuelled greater funding for publishers and made the internet a thriving advertising space, it was inevitably going to spark concern on many fronts. That includes anxiety from users who are becoming less satisfied with trading personal data for free content, as well as unease about data misuse and user rights from global governments, regulators, and tech leaders.
Data privacy legislation, anti-tracking initiatives and the impending demise of Google’s third- party cookie are all consequences of this rising apprehension, as is fragmented data supply. Marketers are simply no longer able to connect the dots from message A to user action B by tracing unique activity — at least not in quite the same way.
But the outlook isn’t entirely gloomy. Advances in artificial intelligence (AI), and particularly machine learning, mean there are now sophisticated predictive modelling options they can use to understand consumer intent and ad impact at scale, without compromising privacy.
How should advertisers adjust their mindset?
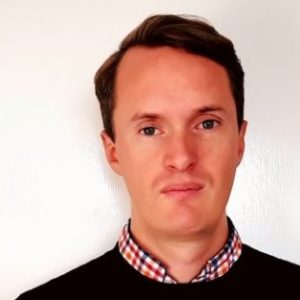
Eoin O'Neill, chief technical officer, Tug
The biggest priority is adopting flexible ways of thinking and working. Much of that will involve emulating channels where agility is the norm, such as SEO. Figuring out which search tactics tip the needle isn’t easy when there are numerous algorithmic variables that could make the difference. As a result, SEO tends to function by testing and learning with machine-learning tools.
By taking a leaf from SEO strategies — leveraging smart tools to run modelling of incoming data — advertisers can gain scaled and actionable insight about user intent, rather than relying on slow, limited, and intensive human categorisation. With deeper micro testing, experts are also expanding their understanding of how Google’s algorithm is working and what it’s likely to do; generating knowledge that can steer search campaigns.
Following this lead and implementing a testing approach supported by predictive analysis will help marketers cut through the noise and determine the impact of their efforts – giving them the wide-ranging insight needed to drive accurate measurement and inform smart choices.
Talk us through the technical details – what does that involve under the hood?
In practical terms, it’s important for marketers to start with the right structure. Simple as it might sound, they should begin by organising tests with a clear idea of what result they want to achieve — and the data they hope to generate. This is critical to ensure consistent control and usability. This, in turn, makes it essential to look beyond purely measuring select conversion goals, such as lead generation, and assessing engagement throughout the user journey.
Doing so enables marketers to obtain data about how users have interacted with their brand at every stage leading to conversion. In the post-cookie age, this will mean increasing use of owned CRM data and first-party data; a move that brings the added bonus of allowing advertisers to get back to exacts (to an extent). As well as providing a clear view of who users are and what actions they have taken, consented data can be fed back into predictive machines: allowing them to evaluate the true impact of certain ads and channels by taking an array of variables into account; looking at outcomes such as overall lead value and quality, or customer lifetime value.
Fundamentally, the goal here is to use first-party data and predictive modelling together. Advertisers can use owned data to supply the basic known input, with machine learning applied in the testing framework to fuel more precise attribution. For instance, modelling may show that despite PPC generating more leads, those produced by paid social were actually more valuable. This is also where optimisation comes in – using such insight to guide future media planning and spending, or at least act as a benchmark for assessing performance.
What will the challenges be, and where are we headed next?
AI’s rising role in marketing evaluation isn’t new, but it’s set to grow as data volumes shrink and leading tech players change measurement tack (see Google’s recent move to axe last-click attribution). To pull alternative insight sources together, handle complex multi-channel campaigns, and make sense of non-click-based activity, advertisers will need sharper tools.
In particular, predictive modelling is poised to take its place as an advertising essential; not only for forecasting, but also looking back to see what has worked. At Tug, for instance, we’re developing machine learning initiatives for user intent modelling and modelled approaches to marketing measurement. These use custom controls for defined testing scenarios or goals, alongside innovative methods for assessing likely outcomes, such as causal modelling.
Obviously, adjusting to the new test and learn approach will have its challenges, as well as barriers to entry. For example, the quantity of statistically significant data required can be difficult to obtain. There is also a certain level of expertise needed, not to mention a high cost of working in this way that could preclude those brands working to tighter budgets. But, most of all many brands and advertisers are simply reluctant to move away from the currently “exact” nature of digital marketing.
However, the automated route is by far the most probable path of change. As machine learning makes its way deeper into the heart of day-to-day measurement and delivery, it will even start to drive activity – fuelling automated decisions, not just informing them.
AgencyDataDigital MarketingMeasurement
Follow ExchangeWire