Smart Cities, Autonomous Vehicles, Artificial General Intelligence Robotics: Q&A with Steve Marsh, GeoSpock
by Sonja Kroll on 16th May 2018 in News
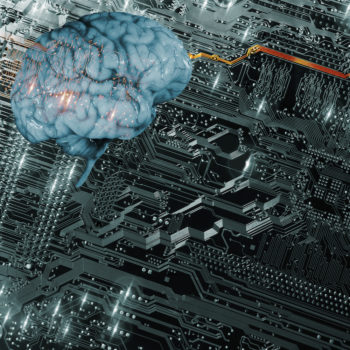
Developments in artificial intelligence (AI) are forming our future world, from advancements in robotics leading us to increasingly human-like AI bots, to an upsurge in focus on the creation of truly autonomous vehicles. However, the question of ethics remains. With increasing technological advancements, it can sometimes seem like there are more questions than answers for the future of AI. Dr Steve Marsh (pictured below), founder and CTO, GeoSpock, explores recent developments, barriers to success, and answers ExchangeWire's questions, with his future vision.
ExchangeWire: It seems at times like the world is split into AI-enthusiasts and AI-sceptics. Do you think AI is a positive step forward for businesses?
Dr Steve Marsh: AI offers many positive opportunities for businesses, not least is its ability to collate, blend, and analyse big data sets and, ultimately, inform smarter decisions. Thanks to the latest advances in computing, it’s possible to harness machine-learning technology and use it to drive cloud-based services, intelligent energy systems, and healthcare innovations such as AI-led surgery. But it’s also important to recognise that AI has flaws, so attitudes towards it can vary: users tend to be either enthusiastic or sceptical. Even expert researchers are aware that AI has the potential to fuel both good and bad outcomes and must be handled with care.
A recent example, where a self-driving car caused a fatality in the U.S., shows that intelligent technology can make serious mistakes. However, what many of the reports failed to explain was that in a scenario where there isn’t enough time to brake, neither humans nor machines can defy the laws of physics – both will fail, yet machines are held to much higher standards than their human creators. Clearly, there is a lesson here that a wider range of training scenarios needs to be factored into autonomous vehicles before they can comfortably beat humans on safety – only then will they become truly viable.
Will we ever get to a point where AI bots think like a human?
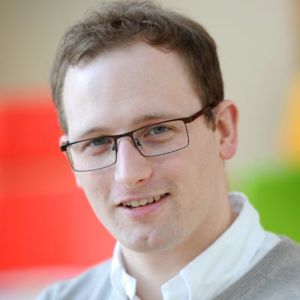
Dr Steve Marsh, Founder & CTO, Geospock
We have already reached a point where machines can replicate many of the activities we undertake as humans. In fact, building a supercomputer that simulates human brain function was part of my Computer Science PhD research and provided the inspiration for an extreme-scale data indexing platform – which provides analytics, builds insight, and enables predictions across space and time – that has evolved to become GeoSpock’s product suite.
Functionally, we can simulate the neurological components – but, when it comes to thinking exactly like a human, machines have some way to go. The current hardware used to accelerate areas such as deep learning (graphics processing units [GPUs] and central processing units [CPUs]) are not particularly well-suited to the way biological neural networks operate. I would say that we are at least 50 years away from a time when machines can replicate an acceptable level of general intelligence within the same power envelope of the human brain, with the main dependency being on the neuroscience rather than the computer science.
So, the question that really ought to be focused on is: why is society so fixated on replicating human abilities, instead of focusing on developing AI that both supports and extends our capabilities? We know that human intellect is not perfect; people are fallible and prone to error. So, as society keeps striving to build bigger and better machines, it seems more logical to set a different objective: developing machines that augment our abilities and mitigate our flaws, allowing us to work more effectively than we ever could before – which I believe can be achieved much, much sooner.
What is currently the biggest barrier to AI succeeding?
I would say the chief obstacle is talent scarcity. While AI has the potential to transform multiple industries, from automotive to retail and smart infrastructure to public services, there is a dearth of individuals with the skills needed to realise its possibilities. The main cause of this exists in the training pipeline; there isn’t enough focus on nurturing interest in the field amongst young talent and current approaches to key subjects, such as mathematics, are too narrow. Schools especially, but also university curriculums, could adapt to focus not just on abstract thinking but also include areas of practical application that takes the fundamental principles taught and anchors it to a real-world example.
Following close behind is the fact that businesses aren’t maximising the potential of data analytics. There is limited understanding of how to collect data in a way that enables easier analysis and insight gathering – which inevitably reduces the wider scope for monetising those insights beyond the primary application, including outside of the organisations. Data silos are a big problem, but also present a big opportunity.
An example of this is in smart cities: if an autonomous city transport firm runs a fleet of cars and uses pick-up and drop-off data to anticipate demand, it may not wish to share the raw data about peak times to a direct competitor, but it could sell high-level congestion insights back to the city authorities, which could then help facilitate better road network optimisations and overall traffic reduction – improving the whole ecosystem as a result.
Does ethics have a place in AI discussions?
Yes, absolutely. It’s crucial to ensure that we continue to utilise the benefits of AI, but do so responsibly. One of the central challenges with AI is that it’s moving from a subject progressed by a small group of specialists to a mainstream technology – and the public is understandably demanding greater clarity and transparency about AI applications.
Recent examples of unethical data usage and the application of AI technology have captured the public’s attention. Leaving aside the unsavoury activities of some businesses, data-driven targeting has been part of internet advertising for years and has long formed an integral element of the value exchange for many websites: in return for free access and services, companies use the resulting data for ads.
But users are now becoming uncomfortable because some companies have historically not been clear on how third parties are deploying their data. The details might be in the website terms and conditions, but perhaps were not clear enough to users, and as a result they can feel a company is not being transparent in its operations. This is something technology firms and leaders need to do better; they must enter into a dialogue with the public that plainly explains how the AI technology is being implemented and what their data will be used for.
The good news is that steps are already being taken to ensure transparent usage of AI and data. In addition to the various new measures being implemented by media giants, collective bodies are forming to guide and boost transparency in AI. Last month, the Nuffield Foundation announced plans to create an institute dedicated to studying the ethical implications of data usage, algorithms, and AI – the Ada Lovelace Institute – which counts the Alan Turing Institute, techUK, and Omidyar Network’s Governance & Citizen Engagement Initiative among its members. So things are certainly moving in the right direction.
Where do you see AI in 10 years?
We can expect to see a dramatic rise in the number of AI use cases for both niche and large-scale purposes. There will be continued adoption and improvement of technologies that bring efficiency to everyday life, such as voice-recognition interfaces and personalised recommendation engines, and a greater adoption of systems such as Alexa and Google Assistant. Self-driving cars will be also be a common feature on our roads, and smart cities will be using autonomous drones and sensors to monitor and improve traffic flow, reduce pollution, and improve living standards.
However, AI tools will not become one self-sustaining, self-aware global intelligence system. In fact, efforts to merge the myriad complex systems would likely reduce their individual effectiveness. There will be convergence at some level. For example, AI systems being aware of another’s parameters so that resources can be distributed evenly. And I think it is also probable that data sharing will increase as businesses and cities reach a mutual point of understanding and, in doing so, improve outcomes all round.
From a business perspective, there will be greater acknowledgement that the data they gather has uses and value beyond the confines of their own organisation. For cities, there will be a realisation that the information gathered by telecoms carriers, Internet of Things (IoT) providers, manufacturing, and mobility services can be collated to help them deliver better services for everyone.
In short, the future of AI will be more collaborative, open, and to the benefit of all.
Follow ExchangeWire