Agentic AI: Beyond the Buzzword
by News
on 20th May 2025 in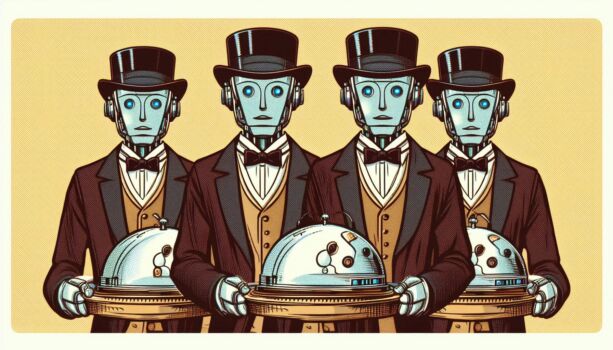
Ad tech has always been a playground for early AI adoption: recommendation engines, bidding algorithms, personalisation layers. But now, something bigger is brewing. With great computing power comes great pressure to build end-to-end ad engines. And that’s exactly why leaders in ad tech are eyeing autonomous, agentic AI. But are we really ready to hand over that kind of control to AI? And is this even that different from the AI we’ve used before? Maybe now we’ll start to get some real answers.
According to Oracle’s The Decision Dilemma, 85% of business leaders have suffered decision distress in just one year: regretting, doubting, or feeling guilty about choices they made. It’s not surprising when you consider they’re facing 10x more decisions than they were three years ago.
But more decisions haven’t meant better outcomes for executives. Instead, they are overwhelmed, underprepared, and plagued by doubt, not for lack of data, but from the excess of it.
In ad tech, the problem is especially acute. A VP managing cross-platform media strategy today has to balance performance lags across walled gardens, manage CPA volatility, evolving regulations, and fielding executive demands, all at once.
What’s missing is a system that can enable autonomous alignment, hold operational memory, and replan execution paths in response to ad engagement. That’s exactly the kind of complexity agentic AI is now being pitched to solve.
Making the leap from reactive AI to agentic intelligence
Unlike Gen AI, which typically waits for inputs and generates fixed responses in a Q&A format, agentic systems are designed to think proactively. They can monitor campaign signals in real time, re-align KPIs, shift spend dynamically, and activate media ops workflows – all without humans in the loop. It’s something Salvatore Nicotra, CIO of Onetag, is already putting to work.
"We use agentic AI to assess traffic patterns in real-time by analysing contextual relevance, historical performance, and advertiser intent to surface the highest-value impressions."
And that’s not easy when ad attributes like viewability or MFA scores change minute by minute and require the system to make smart decisions, fast. "Agentic AI gives us the speed and scale to clean up problematic ads and improve delivery of the impressions that matter for the bidstream."
A potential value maximiser for always-on ad funnels
Ads are no longer planned in neat, linear phases. They're shaped by unpredictable spikes in demand, shifting sentiment, and fast-moving signals across platforms. For marketers, that means constantly recalibrating, often without enough lead time or data.
"The key advantage of agentic AI is its ability to independently generate scenarios that help predict consumer and market response," answers Vivek Kumar, co-founder and CEO of Socialtrait.
His team deploys swarms of 500,000+ autonomous agents to form dynamic AI communities that mirror real-world consumer behaviour. These synthetic groups interact with test content, form opinions, and surface behavioural trends, all before a single dollar is spent on live media.
"It gives us a live testing ground that behaves like the real world, but moves at machine speed, without burning time or budget on traditional research cycles." His customers are already seeing the payoff: higher engagement, lower spend waste, and fewer decisions left hanging in the gap between data and delivery, compared to traditional advertising campaigns.
Vivek’s team is currently using AI to tackle specific parts of the ad funnel. Consumer targeting, to be precise. But where agentic AI really shines is in building a full-funnel system where all components move in sync and serve as dynamic inputs to one another. Instacart, the grocery delivery and pickup service, has already adopted this approach with its universal campaigns.
These AI-built ad engines enable advertisers to run a single campaign under a unified budget. The campaigns continuously optimise across formats based on the overall budget and ad responsiveness to deliver stronger ROAS without constant hand-holding.
What makes agentic AI powerful can also break it
"One of the clearest signs that agentic AI is actually working? It’s when someone says, ‘Hey, can we make sure Evelyn’s in the next meeting?’" says Dustin Engel, CEO of Elegant Disruption. "Using AI is not enough, it should be invited back." But ironically, the biggest blocker to agentic AI success is the very team it’s meant to help.
Rolling out a tool like this without looping in marketers early is asking for chaos. Many still see AI as something that replaces creative control, not enhances it. And when their role shifts from creating to overseeing, resistance is almost inevitable. It’s a pattern we’ve seen before: early adopters are curious and hands-on, especially when the C-suite is involved. But the majority craves stability. They wait. They want governance, a clear chain of ownership, and success metrics to tie their work to.
At Simpli.fi, a platform built around ad success, the real resistance came from adoption. "Our biggest challenges with agentic AI have been around adoption. Teams are still getting comfortable with the idea of agents not just assisting, but acting," admits Peter Luttrell, VP of AI implementations. "Building trust takes time, especially in workflows tied to customer experience or budgets."
Even when your team’s bought in, the technical fragility of your infrastructure can quietly hold agentic AI back. It needs clean, connected data to function well. But in most organisations, that’s far from the reality. If your agent is managing ad performance across Meta and Google, but sales data is buried in a legacy ERP, loyalty signals live in a separate CRM, and there’s no unified API, your AI might start flying blind, or worse, hallucinate.
It’ll fall back on weekly stitched-together reports and make decisions based on stale, incomplete information. That’s how the budget ends up getting funnelled into underperforming campaigns, while strong customer signals get ignored. As Peter rightly says, "Most systems were never architected with agents in mind. So plugging them into fragmented tools adds friction."
Towards agentic AI resilience
Success with agentic AI is picking up across the advertising world. What stands out is how the most forward-looking teams are shifting their view of it. They no longer see it as a tool for isolated automation tasks, but as a persistent, evolving teammate.
"Our advice is to focus on small, purpose-built projects," Peter shares on how to find success with pilot agentic AI projects. "Cross-functional teams deploy minimum viable agents designed to tackle specific bottlenecks or inefficiencies. They’re helping us accelerate creative QA, reconcile insertion orders, and bridge gaps between siloed systems."
For Dustin, the shift is happening inside collaborative workspaces. "For true success, we should think about using agentic AI as a kind of strategic sidekick," Dustin says. His team is now successfully building autonomous tools that support workshops, planning sessions, and innovation sprints.
"It’s great when you’re juggling multiple teams and can’t be everywhere at once. We've seen projects move from vague ideas to clear decisions in far less time. Clients with less experience in structured strategy work feel more confident when they have our AI agents there to back them up.”
To scale that impact, leaders must tune into how teams interact with the agents. "The right way to use and scale AI is to continuously ask: Are people refining the agent’s output? Are they giving feedback, requesting improvements?" Peter says. "We will see success not just in what the agents do, but in how people respond to them. Specifically, we will have to assess whether teams trust them, challenge them, and ultimately build with them."
Follow ExchangeWire