Open Machine Learning: A Request to the Digital Industry
by Lindsay Rowntree on 17th Nov 2016 in News
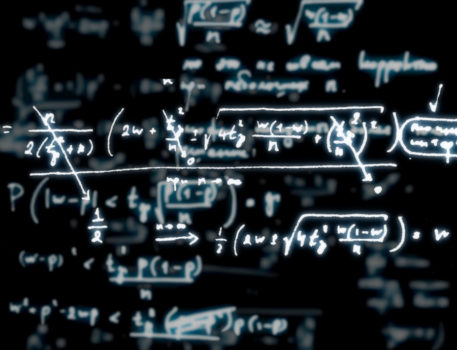
At ATS New York 2016, Nathan Woodman, general manager, Demand Solutions, IPONWEB delivered a keynote speech on the topic of first-party machine learning in programmatic, with a request to the industry: can we create a framework for ‘Open Machine Learning’?
Data, data, data
With data growing at an unprecedented rate, we exist in a situation of extreme complexity; and the most complex model far exceeds human comprehension, with 50 million potential outcomes. Everyday we are creating 2.5 quintillion bytes of data, with more people connected to the internet than ever before, more connected devices with more sensors, and the meteoric rise in programatic and RTB adding more marketing-related data – it’s adding up fast. IDC estimates the amount of digital data being collected will grow from 4.4 zettabytes in 2013 to 180 zettabytes in 2025, with one zettabyte being the equivalent of one trillion gigabytes. “So we’re talking 180 trillion gigabytes’ worth of data by 2025”, explained Woodman. “That’s what people really mean when they say Big Data.”
According to Forrester: “There’s too much data. Marketing departments can’t deliver the analytics and deploy that level of agility that customers require. We’re reaching the limits of human cognitive power.” However, Woodman believes we’re already far beyond that: “A machine is infinitely superior when it comes to processing and applying information. Data is only really valuable if you can use it to identify patterns and make decisions about who to target, with what creative, in what context, and at what price, in real time”, explained Woodman. “When we’re talking about zettabytes of data, that task is simply beyond the realm of human comprehension and capability.”
Enter machine learning
This is where machine learning comes to the fore. The level of complexity that exists in machine learning is within three fields: Data, Analytics, and Machine Learning. Analysis involves processing the data for human consumption: “It dumbs it down into enough variables to be understood by a human”, explained Woodman. “Machine learning doesn’t dumb down data, it just applies it to a goal. We don’t know what the machine is doing, we just know it’s achieving its goal.” Machine learning looks for patterns among massive data sets, uncovers hidden insights, constructs algorithms to make data-driven predictions or decisions, acts in real time, and grows and changes when exposed to new data. While around since the 1950s, it’s only really gaining popularity today because of the vast swathes of data we have available.
Machine learning is already redefining digital advertising, and programmatic specifically. According to Juniper Research, USD$3.5bn (£2.4bn) is being funnelled into machine learning today and that is set to increase to a phenomenal USD$42bn (£28.7bn) in 2021.
A brand's competitive advantage
Referring to the running theme throughout ATS New York of 'Bring Your Own Algorithm' and how brands should be building their own, Woodman explained the importance of machine learning to a brand’s competitive advantage: “Brands need to have their own machine learning and not use somebody else’s.” And, according to Woodman, there already exists a number of companies able to build first-party machine learning models on behalf of their clients, citing IBM Watson, TensorFlow, and PredictionIO as the key players in this space. Woodman explained that machine learning and algorithms are currently owned by the walled gardens. Brands are currently giving all of their data to the likes of Google and Facebook, who are using it to build machine learning systems – despite the data belonging to the brands, the walled gardens own the black box responsible for their performance. “If brands are in charge of their own machine learning”, explained Woodman, “performance may be inferior, but it becomes their black box.”
What is the real competitive advantage of first-party machine learning? According to Woodman, the benefits are obvious and it is possible, and necessary, for brands to fully adopt machine learning systems. It brings unique first-party audience and media data into decisioning rules, it executes to a brand’s custom or blended KPI, and it modifies that KPI as the machine learns what works and what doesn’t, creating distinct derivative attributes based on the brand’s knowledge of target segments and also avoids fraud by seeking custom, hard-to-game KPIs.
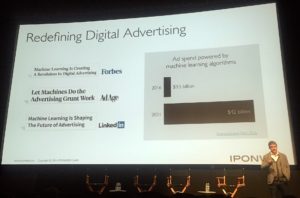
Nathan Woodman, General Manager, Demand Solutions, IPONWEB
“At this point, machine learning is mission-critical for marketers”, said Woodman. “That’s why you see major players like Google, IBM, and Apache entering the field and providing open tool kits that are empowering brands to deploy machine learning within their enterprises in a variety of ways.” Even DSPs are entering the space, offering their own form of programmable machine learning tools, such as AppNexus’ Bonsai decision trees or The Trade Desk’s bid multipliers – these players are empowering brands to develop first-party machine learning algorithms that leverage their own unique data sets and KPIs to buy media programmatically. However, this still brings its challenges in the form of closed implementation. “It’s very much a closed ecosystem”, explained Woodman. “The algorithms you’re able to build in one DSP are closed and not portable. What you build in one DSP can’t be used in another.”
Open Machine Learning
This is where a request for Open Machine Learning comes into play. “We think the market is ripe and needs Open Machine Learning”, said Woodman. “It’s similar to what OpenRTB did for the bid stream. There is a need for a machine learning protocol – a superior class of learning model – for the industry to capture the potential USD$42bn (£28.7bn) of machine learning spend in the future.”
Woodman is attempting to socialise an idea: “We’re putting the idea out there and want the industry to adopt it. It would be a model output allowing algorithms to be transported across DSPs. The closest we have now exists in the statistical science space – a markup language called PMML, which can be an output from tools like SPSS.” According to Woodman, it’s not as advanced, but there are certain types of protocol in the statistics industry, which could be modified to handle a complex class that works in the programmatic space.
If the industry is keen to adopt this, it won’t happen overnight. Woodman explains that if the concept of Open Machine Learning sticks, it will take three to four years to adopt and the enthusiasm with which brands embrace machine learning will depend on its transferability across multiple systems. “To receive a chunk of that USD$42bn (£28.7bn) in ad spend, the industry needs an open environment for brands to embrace this approach.”
Follow ExchangeWire