Exploring Bias in AI: How Can We Overcome It?
by News
on 1st May 2024 in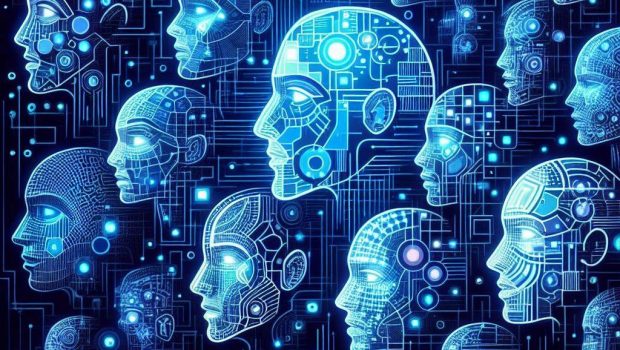
We explore the gender and racial biases in artificial intelligence (AI) systems, from YouTube’s automatic caption generator to models built to predict liver disease and diagnose skin cancer. Why do these biases exist, and how can we work around them?
How Do Biases Show Up in AI?
Voice Recognition and Name Screening
Have you ever struggled to get a voice recognition device to understand you? If you are a woman, this wouldn’t be so surprising: studies have found that women’s voices are recognised with lower accuracy than men’s by voice recognition systems. Voice recognition systems have demonstrated bias when detecting the voices of speakers across different genders, accents, and races.
A study which examined YouTube’s automatic caption generator found that word error rate was higher for women than men. It demonstrated the caption generator’s accuracy also varied with dialect: speakers from Scotland produced a much higher rate of error than those from the United States or New Zealand, for example. In no dialect was accuracy more reliable for women than men.
Another study which investigated automatic speech recognition systems from Amazon, Apple, Google, IBM, and Microsoft, found racial disparities. Their testing produced an average word error rate of 0.35 for black speakers, compared to 0.19 for white speakers.
More specifically, AI has been found to discriminate against candidates based on their names, favouring some demographics over others in certain circumstances. This bias is present to an extent which would fail benchmarks used to protect candidates from job discrimination. With voice recognition systems being used regularly throughout companies’ recruitment efforts as well as for other important processes such as residence applications, the tools’ shortcomings become serious. Beyond affecting someone’s experience using Alexa or Siri for personal use, these biases can affect people’s job prospects or whether they are granted the right to remain in a country.
Facial Recognition
When it comes to facial recognition, the poorest accuracy has been found on black women. In a 2018 project, Gender Shades, researchers investigated the accuracy of four gender classification algorithms, including those developed by IBM and Microsoft. Participants were grouped into four categories: darker-skinned women, darker-skinned men, lighter-skinned women, and lighter-skinned men. All algorithms performed the best on male versus female faces, with an error rate difference of up to 20.6%. They all also performed best on lighter versus darker-skinned faces, with a difference in error rate of up to 19.2%. While lighter-skinned male faces achieved an error rate of only 0.8%, darker-skinned female faces reached an error rate of up to 34.7%.
In a world with increasing public surveillance, biases in facial recognition also present a potential to exert harm among marginalised groups. So far, people’s false identification through facial recognition systems has led to wrongful arrests and convictions.
Social Media Algorithms
Social media is not free from bias either: analysis has found the objectification of women embedded into their algorithms. An investigation by The Guardian found that social media algorithms unnecessarily censor and suppress the reach of photos featuring women. In order to keep users safe online, AI is used to detect inappropriate content – however, women’s and men’s bodies are not treated the same way by the algorithm. Images of women are generally classified as more “racy” than comparable ones of men. Often, women’s bodies in everyday situations – such as exercising or at the beach – are tagged by AI as sexually suggestive. Consequently, this can result in content being suppressed without the user being notified, and users getting shadowbanned by platforms.
Ad Serving
Similarly, biased AI has caused trouble in advertising. A study conducted in 2021 which investigated Facebook’s ad delivery system found that different jobs were displayed to men and women, despite requiring the same qualifications and not specifying a particular audience on the basis of demographic information. The findings suggested that Facebook’s algorithm was picking up on the current demographic distribution of the jobs in question. Since, Facebook has been involved in legal action over its “discriminatory” ad algorithms.
Biased AI can prevent consumers from being exposed to certain information or brands. With a large number of advertisers harnessing AI to determine which ads are shown to users, there is a danger that biased algorithms can alienate particular audiences. As a result of poor quality training data, improper model deployments, or analytics methods, among other variables, marketing models can reflect prejudice, stereotyping, or favouritism towards a particular group of customers. By failing to address the issue of biased algorithms in their tech, advertisers risk a revenue loss.
Image Creation
AI image creators also demonstrate society’s biases, for example when depicting certain professions. Testing this out myself, I instructed an image creator to produce a realistic image of a doctor. All of the potential images I was presented with appeared to be of male doctors. When asked to create a realistic image of a nurse, all options presented to me contained nurses which appeared female.
Experimenting further, I found that the tool’s image creation also reflected existing gender stereotypes surrounding male/female emotions. Upon requesting an “emotional person”, I was presented with images which appeared to show women. Likewise, the request to see “a nurturing person” led me to images of women warmly embracing young children.
Medical AI
More alarmingly, many biases have been found in AI used for medical purposes. Research by UCL found that AI models built to predict liver disease from blood tests were twice as likely to miss disease in women than men. Similarly, there have been worries that heart disease could go underdiagnosed among women when using AI systems to read chest x-rays – these systems are predominantly trained on images of men, who generally have larger lung capacities.
Research also suggests that the AI used to diagnose skin cancer is less accurate for those with darker skin. As well as AI models being trained mainly on images of men, clinical images are also often of white skin. In 2016, researchers in Germany developed an AI model to detect melanomas – although it was hailed as a promising example of AI’s potential in the medical field, more than 95% of the images used to train it depicted white skin, suggesting considerable room for error when using the technology on those with darker skin tones.
A Lack of Diversity
While women make up around half of the global workforce, they only make up 30% of the world’s AI workforce. In both the UK and US, women make up just over 20% of the data and labour AI market. With AI tech being predominantly developed by men, many gender biases go undetected during the testing process. Similarly, graduates who obtain AI PHDs in the US are predominantly white: figures show that in 2019, only 1.6% were multiracial, 2.4% Black or African American, 3.2% Hispanic, and 22.4% Asian (versus 45.6% white, and 24.8% unknown).
Moreover, many of the datasets being used to develop AI tech are predominantly male-focused. Voice recognition systems, for example, are trained mainly on the voices of men – this is why higher pitched speech, more typical of women’s speech, has been found to be more difficult for automatic speech recognition systems. Datasets used to train AI are also often dominated by data of white people, as shown through the AI model designed to detect melanomas. Unsurprisingly, biased data sets result in biased algorithms and tools. Largely, the biases in AI used for personal and professional use, as well as in the medical field, are the result of not enough diverse involvement in both the creation and training of AI models.
How Can We Fight the Bias in AI?
The examples listed are just some of the many which exist – how can we work to tackle them? Firstly, people need to be aware of them and understand why they exist. If we approach AI knowing that it may reflect certain biases found in society, we are more effectively able to prevent the tool from playing these out. In certain cases, using specific instructions which demand diversified results can train the tools we are using to be more diverse.
On a larger scale, ensuring that data collection is sufficiently diverse should be the first step. This will be made more likely by diversifying the AI workforce; a diverse AI industry will be incredibly important in working to tackle these biases. Companies should also be transparent about their data, as well as about any known shortcoming their AI models/tools may have. Many companies’ lack of transparency around this worsens the harm caused. AI can certainly be used as a tool to do good, but currently, human involvement remains vital to ensure such biases are detected.
AIDiversityVoice assistantYouTube
Follow ExchangeWire