Why You Must Clean-Up Your Stores of Unstructured Data: Q&A with Des McHugh, ZL Technologies
by Lindsay Rowntree on 27th Sep 2017 in News
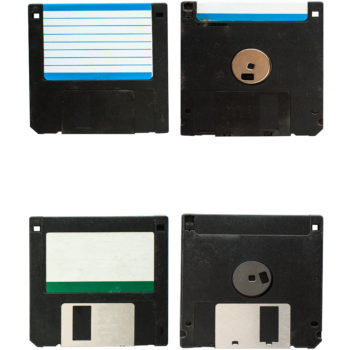
Have you heard of the term 'unstructured data'? If you're currently in the throes of preparing your company for the looming General Data Protection Regulation (GDPR), then it's probably a term you should become familiar with, as it applies to more than you may think. Des McHugh, information governance consultant, ZL Technologies (Ireland), outlines for ExchangeWire exactly what it is and how it must be handled to ensure GDPR-compliance.
ExchangeWire: What is 'unstructured data'?
Des McHugh: In formal terms, unstructured data refers to data that does not have a predefined data model; whereas structured data has a predefined model and, therefore, can be stored in a database with a defined structure.
In human terms, unstructured data is primarily electronic communications – email, instant messages, social media posts, and files and other documents. These data types exist in communication systems such as email and messaging servers (and inboxes) and in file stores such as network file shares and collaboration or content management systems.
What is the requirement of handling unstructured data stores to ensure GDPR-compliance?
GDPR is concerned with personal data and is mostly unconcerned with what content type or format in which that data exists.
Organisations must have a lawful basis for processing personal data and must adhere to strict protection and control principles to protect the fundamental rights and freedoms of the individual, as per the European Convention on Human Rights.
This means that personal data must be stored in a minimised manner – only store the minimum set of fields that are needed, for the minimal number of data subjects, and for the minimal period of time. Furthermore, if an individual wants to know what data is being processed about them, you must be able to tell them and to furnish their data to them, as well as correct, or possibly delete, on request.
Whatever is stored needs to be secured and access should be controlled as tightly as possible, while still allowing the desired processing to happen.
To accomplish all this, there are certain challenges that need to be overcome.
You need to be able to analyse the email, messaging, and file stores to find any personal data being stored there. Once found, you need to be able to delete what is not needed, and apply the desired control and governance policies on what needs to be retained.
You need to be able to apply the necessary retention policies within that governance so that personal data is not stored beyond its intended life.
You need to be able to find data related to specific individuals when they do come looking for access, rectification, or deletion. Once found, you also need to be able to review in case there are reasons why you should not produce it. Depending on this review, you might need to withhold or redact before production, e.g., because of an ongoing litigation or investigation, or because of the existence of personal data of other individuals in the content.
Finally, if they do request access to their data, you need to be able to produce it for them in, most likely, consumable electronic formats.
How would a business know what unstructured data it has?
It should be very easy to know what unstructured data stores an organisation has, but knowing what is in these stores is the big challenge.
For GDPR purposes, you need to be able to scan and analyse the content in all these stores to find the personal data within. There is, unfortunately, no magic bullet for identifying personal data, particularly in an unstructured store that does not have a data model.
A structured store, such as a database, has a data model so it is mostly possible to identify personal data at the field-level. For example, if a field is called 'Name', then it is likely that all the contents of that field are names and hence personal data.
In the world of unstructured data, it is less simple. Certain fields and types of personal data can be identified with certainty, e.g. Social Security numbers. However, fields like dates of birth are indistinguishable from regular dates and therefore need some form of context interpretation.
Techniques to identify personal data in unstructured data range from regular expression patterns, word searches including wild cards, stems and fuzzy matching, all the way up to machine-learning-type methodologies. With this type of advanced technique, engines can learn from a sample set of content to flag probability or risk scores on a wider data set.
When analysing the unstructured content to find the personal data, advantages can be gained from techniques that allow high-level or sampling analyses that enable identification of 'hotspots' where personal data is concentrated. Granular control of further analysis can then be applied to enable an efficient search and analysis process that achieves maximum discovery with minimum effort.
How would a business begin to clean up its store of unstructured data?
Clean up, or remediation, of the unstructured stores is dependent on the analysis described above. You can’t take action on content if you don’t know what it is.
The identification of ROT (redundant, outdated, and trivial data) is an important part of the process. A significant amount of any unstructured data store will be ROT and a significant subset of this will be identifiable by metadata alone, i.e., can be classified and remediated without doing unnecessary 'heavy lifting' in the form of content analysis.
Removal of this ROT, therefore, can significantly reduce the volumes that need the expensive content analysis, and in fact most likely pays for itself in terms of effort and time savings.
When performing content analysis on the remaining data, some relatively easy extensions of the techniques used to identify personal data can be used to also classify other content.
Remediation can then be applied on the different classifications of data. Further ROT can be deleted. Business value data can be archived or flagged as records. Sensitive data can be quarantined or moved to enable stricter control. Lastly, personal data can be deleted, if not legitimately required to be retained, and can be quarantined or moved to enable the stricter control if it does need to be kept.
Would this be a costly process? Would it require additional resources/systems to identify and clean unstructured data stores?
How long is a piece of string? The costs will be driven by the volume of data existing in these systems and stores, and the level of confidence that the organisation wants to attain in remediating personal data on their systems.
It also very much depends on the organisation’s starting point. Do they already have robust information governance tools and policies? Do they already have a tool that can perform content analysis on unstructured data? Just in files, or in email and messaging stores also? Social Media? One tool that can analyse all, or are they currently equipped with multiple tools for different systems that have different capabilities and different views and techniques?
Do they want to do a one-off clean-up job, or do they want to implement a robust information governance strategy on their ongoing content creation? Do they want this strategy to encompass not just information management and compliance, but be interwoven with their legal e-discovery processes and their records management capabilities also?
The simple answer is that all of the above needs to be considered – and the larger the organisation, the more of the above they will need to incorporate into their strategy. The more that they need to encompass, the more likely that they will need dedicated new tools and programs. Some of the new tools and systems can also pay back very quickly, in terms of the value that can be utilised over simpler or more brute-force technologies.
It also has to be remembered that there are levels of return on investment in this that pay above and beyond GDPR. Robust information governance unlocks value for ongoing business and simple data clean-ups deliver returns in terms of storage costs and backup and recovery times.
What if businesses aren’t prepared for this in time?
This is a real concern, as we are now very short on time to get ready. The good news is that GDPR is about managing risk rather than compliance. Projects, programs, and strategies that are in train, even if incomplete, come 25 May next year, will contribute both to the ability to be as compliant as possible and will mitigate any sanctions that are applied in the event of breaches of the regulation. The Data Protection Authorities will be much more impressed by an organisation that has a robust and defined strategy than an organisation that has tried to get away with a 'tick the box' approach.
Follow ExchangeWire