A Powerful Ad Buying Tool: Maximising Programmatic Direct Performance
by Lindsay Rowntree on 1st Dec 2017 in News
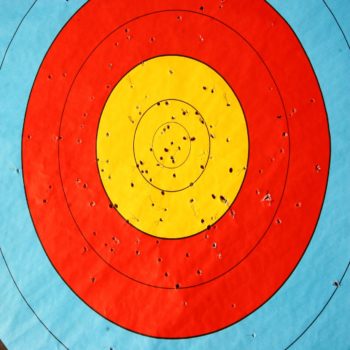
In association with OpenX
Speed or certainty – when programmatic first emerged, buyers had to choose between the two. They could opt for real-time bidding (RTB) and large-scale efficiency, or traditional direct deals and guarantees of inventory. It’s not surprising that the arrival of programmatic direct, which combines the efficiencies of programmatic with the certainty of buying from approved partners, has been enthusiastically embraced by buyers and publishers alike. In fact, OpenX has seen their programmatic direct revenue alone rise by 500% since 2016.
One of the key considerations for advertisers using programmatic direct models – such as private marketplaces – is what data types to leverage for the best campaign performance. It’s imperative that they understand the types of data available and consider how to most effectively reach the right audiences.
Richard Kidd, VP, head of business development, EMEA at OpenX, reviews the two main types of targeting data: behavioural and profile.
Behavioural data
In brief, behavioural data can be defined as information relating to past consumer activity, and subsequent inferences about needs, wants, and future intent. It consists of multiple content-consumption data sources (such as site visits, video views, ad clicks, app usage, and social media interactions) blended to create a detailed view of what individual users do. And, the data itself is acquired via tracking: demand-side platform (DSP) or data management platform (DMP) tags served by advertisers and publishers; mobile environment, proximity (location), and general insight data usually comes from device IDs, not cookies.
The unique first-party insight this offers marketers can be highly valuable. For instance, if a user frequently visits certain sites, especially retail or brand sites, this can be a strong signal that they are preparing to buy. Thus, marketers have the ideal basis for serving tailored messages that reach users at the ideal moment to drive purchases.
Behavioural data, however, has its limitations. First-party data typically covers a small section of target audiences, particularly proximity data – which only includes users who freely share their device location. The result is that, while returns generated by such data are high, the reach of targeting or retargeting campaigns leaves something to be desired.
Another type of behavioural data is third-party segment data. It categorises users based on interests, and is often combined with first-party behavioural data to boost the reach and targeting of a campaign. Although these data packages do provide a broader insight range, their value is often lower because retailers regularly retain the best data for their own marketing purposes. As a result, in-market segments are chiefly composed of user interactions with entertainment or news sites from which interests are inferred, rather than brand sites, which provide a more direct correlation of interests and individual purchase intent.
Behavioural data used by itself is further limited by the fact that marketers don’t have a clear picture of interaction context, such as where users consume content and their interests outside digital content.
To make the most of behavioural data, marketers should, therefore, consider following these key steps:
Varied buys: By purchasing from selling environments with a wide range of ad space, such as targeted exchanges and multi-publisher private marketplaces (PMPs), marketers can ensure they realise the full potential of first-party data. For example, high-quality behavioural data can be used with RTB engines or high viewability deals to identify and secure the best matches.
Selective targeting: To maximise returns and relevance, marketers can apply filters that remove site visitors from their targeting list who are less likely to buy from their brand. For instance, users who have just booked a holiday could be excluded from holiday sale offers.
A data cocktail: Once third-party data has been meticulously assessed, the most appropriate segments can be enhanced by the addition of other insight sources that allow marketers to determine whether clicks or site visits are intentional, or not, such as profile data.
Profile data
Unlike transient behavioural data, profile insight is based on tangible characteristics, such as consumer age, gender, ethnicity, and product ownership. Generally speaking, it is obtained in one of two ways: when users fill out digital registration forms on brand sites and apps, or by connecting offline profile information (household data) with device IDs and cookies.
The chief attraction of profile data is its accuracy. Not only are the user attributes it includes less mutable than behavioural data, but it is also drawn from more reliable sources – like voter registrations, censuses, and home-ownership records. For marketers, this makes it a valuable source for targeting.
Yet, profile data also has its limitations. Information, for example, is not as quickly accessible: the process of joining offline profile data with device IDs and conveying the resulting insight to programmatic platforms can be lengthy, taking a week on average. What’s more, this procedure is further hampered by the non-addressability of many online cookies. Only 30-45% of cookies generated outside walled gardens – where users are authenticated by logins – can be successfully linked to offline profiles. And, even when relevant users are identified, there is no guarantee that they are immediately ready to buy.
Getting the full user view
In isolation, profile data has great inherent value, but restricted utility. Consequently, the most effective way to deploy it is as one half of a bigger insight puzzle, the other being third-party contextual data. For instance, marketers could serve ads for sustainable cars to users who often visit the brand site, support environmental causes, and own other related items.
The best way of achieving this depends on marketers’ goals. They can fuse profile and behavioural-category targeting when buying inventory to achieve optimal relevance and scale, be that through PMPs or real-time guaranteed channels.
Alternatively, they can cross-reference key audience segments to improve targeting accuracy by connecting profile data with third-party in-market data for larger PMP and exchange deals – thereby excluding any individuals who do not align with required profile attributes.
We’ve covered some recommendations and best practices that will help marketers create the ideal blend of data. But it’s also crucial not to forget quality. Before joining forces with data or technology providers, they need to ensure partners have robust quality control measures by asking essential questions. Where and how is data being obtained? Is it modelled or probabilistic? How fresh is the data?
Once partners are chosen, marketers should continue their quality watch: randomly reviewing data records and assessing vendor performance individually and comparatively. Campaigns can be divided into an array of line items and targeted using data from different partners. Last-touch attribution, for instance, tends to allocate all the credit for a conversion to the last ad seen; so it is better to measure against a non-targeted control group, too.
By carefully using the right mix of behavioural and profile insight in programmatic direct campaigns, marketers can wield a powerful ad-buying tool that marries the best of direct buys with the programmatic future.
AdvertiserAutomated GuaranteedPMP
Follow ExchangeWire