6 Reasons to Switch from A/B Testing to Always-On Experimentation
by Lindsay Rowntree on 13th Mar 2019 in News
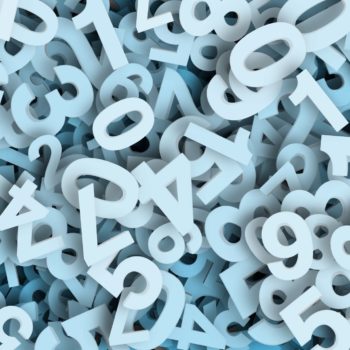
Experimentation is the tool of choice when trying to learn about outcomes of actions that cannot be calculated or forecasted.
In digital marketing the most obvious use cases for experimentation are: 1) exploring new channels or trends, like new social media platforms, publishers or formats; 2) optimising campaigns by gaining a better understanding of which combinations of campaign elements, personalisation, and audiences work best; 3) testing new products, strategies, business models, or pricing strategies with smaller audiences before rolling them out broadly.
While many organisations have migrated from traditional 'waterfall' methods to agile workflows for product development, it seems difficult to apply a more iterative process to campaign optimisation workflows or channel adoption, for example. The common industry practice, that leverages A/B significance tests for experimentation, comes with quite a few flaws that make the switch to an agile process impractical.
The key issues that prevent a shift to an always-on experimentation process, which would enable companies to iterate, optimise, and innovate faster by constantly learning from continuous marketing experiments, are:
1. Prior setup required
Significance tests require the specification of significance level, power, and effect size, which are often complicated to determine before running the test.
2. No test peeking
Significance tests require a certain, pre-defined number of observations to become conclusive. You can’t look at the results before the end of the test period; which in turn leads to long test periods, even if the result is clear early on.
3. Limited number of variations
Testing more than two variations (A/B/n or multi-variant testing) requires many additional observations to become significant, which leads to even longer test periods.
4. Inconclusive results
Inconclusive test results happen regularly and require the test to be re-run with different parameters from scratch, which wastes time and resources.
5. No quantification of test results
The result of a significance test will only confirm that the size of the tested-for effect is probable, but it could also be greater – it won’t determine how big the real effect is.
6. Resource dependencies
To setup and analyse A/B tests, marketers usually have to depend on restricted external or internal data science resources.
All of these flaws aside, significance tests have a few strengths: they are manageable, easy to teach, and inexpensive to compute. Trying to scale and automate them to adopt an always-on experimentation process is not feasible, though.
Advancements in data science, and the ability to crunch large data at affordable cost, have brought alternatives to significance tests to the table; namely Bayesian inference, a method of statistical inference in which Bayes' theorem is used to update the probability for a hypothesis as more evidence or information becomes available.
With Bayesian inference, it’s possible to specify how much better a particular variation performs, compared with another variation, as well as compare the performance of all variations at once, rather than analysing them in pairs. The ability to break the data down in different ways, without the need for statistical corrections, enables a more exploratory process – rather than getting confirmation using classical frequentist approaches, which would comprise repeated sampling to produce similar datasets as have already been gleaned.
First solutions are entering the market
Key leaders in the industry are switching to this approach and the first products are hitting the market.
Quantify, a solution released by marketing intelligence platform Admetrics, claims to deliver on all of these promises. By combining machine learning with Bayesian statistical modelling, it simplifies the setup and analysis of experiments, so marketing teams can carry out and analyse experiments on their own and take full control of their experimentation roadmap.
As outlined and published in Admetrics’ white paper, Quantify requires 60-90% less data than traditional test methods to generate conclusive results. According to the company, the solution has no limitations to the number of variations which can be tested at the same time, allows test peeking, provides meaningful estimates of metrics and effect sizes from day one, and allows marketing teams to easily switch to always-on experimentation.
Admetrics CEO Markus Repetschnig thinks that always-on experimentation is a true game-changer: “Quantify does not only speed up decision-making processes; it opens up a whole new spectrum of possibilities by empowering any marketer with abilities that have only been available to experienced data scientists so far. Experimentation can now become an integral part of any marketing strategy.”
It will be interesting to see how quickly a new, more agile and automated process like always-on experimentation will get adopted, as the combination of AI and process automation has the potential to change marketing substantially.
Follow ExchangeWire